Enabling end-to-end Automation in Analysis and Reporting
PHUSE US Single Day Event 21-MAY-2021
Summary
The PHUSE US Spring 2021 SDE was an interesting cross-section of perspectives on automation of Analysis and Reporting. My takeaway messages were:
- End-to-end machine readable standards are still a work-in-progress, and there is not a clear path to standardisation around e-Protocol and e-SAP
- CDISC aim to make it simpler to implement software that automates standards (rather than publishing standards as ‘600-page pdf documents’); this is based on the proprietary CDISC Library
- Key implementation challenges include: Managing change, Budgets (it takes longer than you think) and Silo business processes
- PHUSE have a mature set of safety reporting deliverables, including SAP definitions, statistical methods and visualizations. This can provide a solid basis for automation projects.
DISCLAIMER: I missed one session by Farha Feroze (Symbiance) on Automated TFL Moch Shell Generation using AI Techniques (ML/NL), so is not included in this report!
Future State
The day was (excellently!) chaired by Bhavin Busa, and Gurubaran Veeravel, who kicked-off with reference to the CDISC 360 ‘Future State – Analysis and Reporting’, noting that “we are not there yet!”
This is a future-state vision that is based on automating the current reporting pipeline. Currently ADaM and TFL programs are written manually.
CDISC Standards
Anthony Chow presented an overview of the CDISC Library, and current/future projects related to Analysis & Reporting
CDISC aim to make it simpler to build software that automates standards-based processes.
- The CDISC Library provides an application programming interface (API) to ‘normative metadata’ on all CDISC standards
- CDISC is working with open source projects on tools to access CDISC Library
- CDISC have Analysis & Results projects ongoing and about to start recruiting members: MACE+ project, Safety User Guide, and Analysis Results (ARM) Standard development.
Path to Automation
Andre Couturier & Dante Di Tommaso (Sanofi) gave an insightful presentation on the challenges facing when implementing end-to-end automation (for a start.. don’t call it automation!)
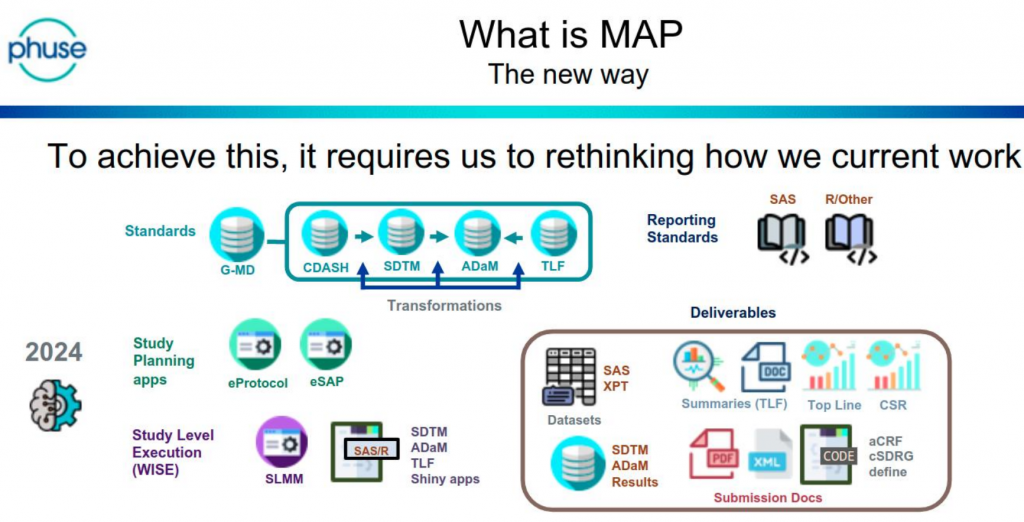
- It is key to communicate a clear vision and ‘sell upwards’
- Key challenges include change management, Silo business processes, and budget (it takes longer than you think!)
- Decide whether existing processes are ‘Deterministic’ or ‘Intelligent’ – Deterministic processes are candidates for automation, and Intelligent processes can be ‘facilitated’
- Sanofi use the acronym MAP (Metadata Assisted Programming) to describe the change in approach
Safety Reporting
Mary Nilsson (Eli Lilly) provided a comprehensive overview of the work that PHUSE has done on Safety Reporting. A comprehensive set of deliverables are available phuse.global
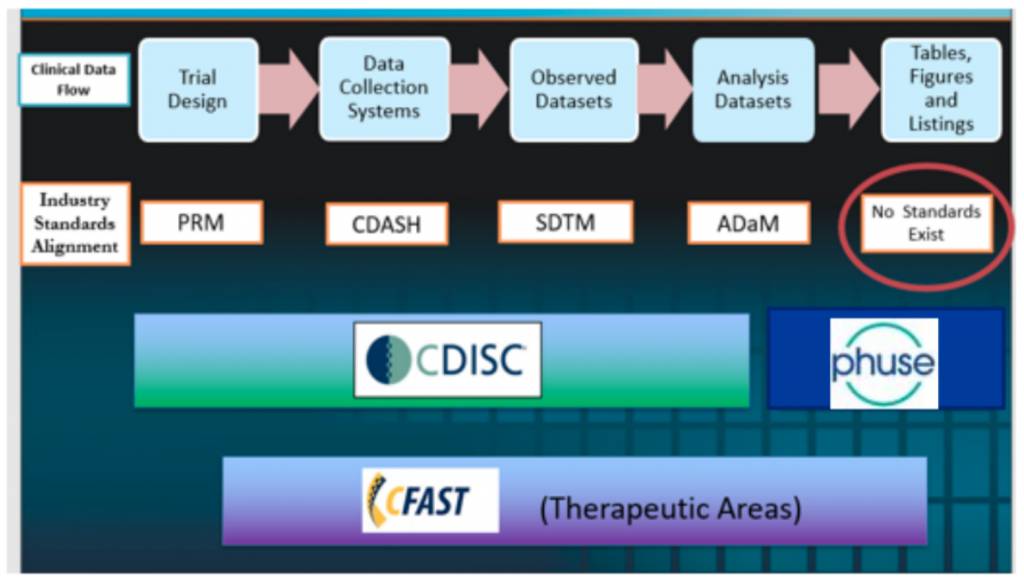
- The two working groups are: Standard Analyses and Code Sharing (pre-2020) and also Safety Analytics (post-2020)
- In addition there are training videos covering pooling data, safety analytics and for integrated reporting
- The deliverables include SAP text, statistical methods, and visualisations
- This is a body of knowledge that can provide a solid set of ‘source documentation’ for automation projects
Traceability and dependency
Gurubaran Veeravel walked-through how Merck perform impact analysis when standards change – specifically to determine program dependencies and variables used.
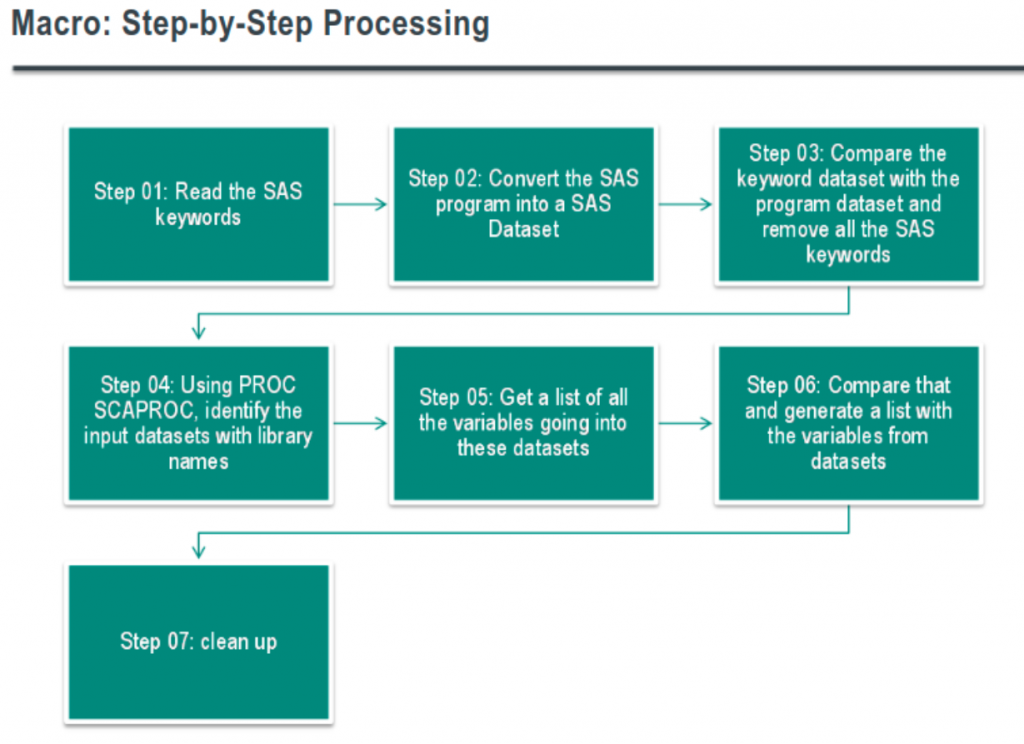
R demo – TFL Generation
Jem Chang & Vikram Karasala (AstraZeneca) presented how R programs can create RTF outputs with the same layout as existing (SAS) outputs. Alternative/existing R packages are available, and the pros/cons of each were discussed.
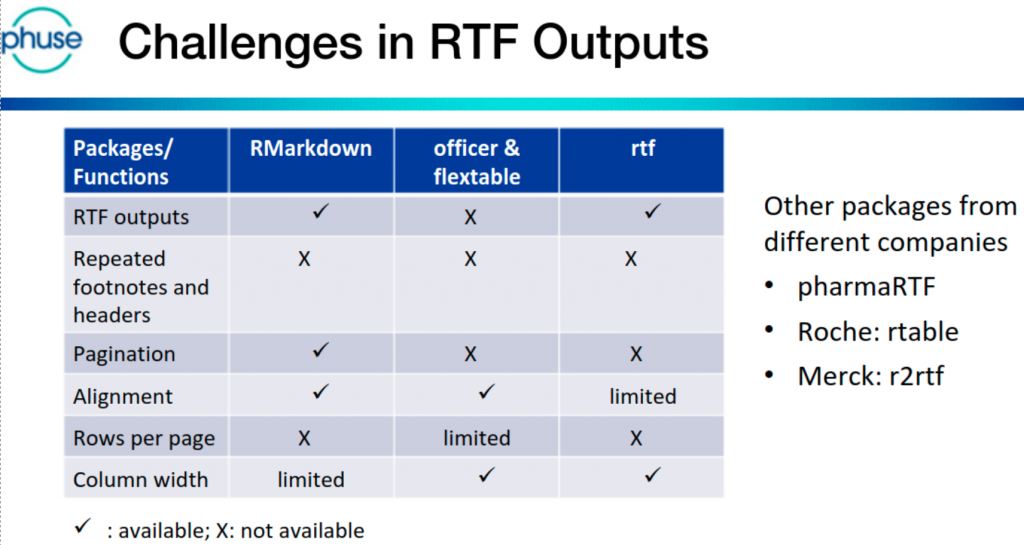