Behold, the people is one, and they have all one language… and now nothing will be restrained from them, which they have imagined to do.
Genesis 11:6
A compelling vision
Clinical Trial analysis and reporting is changing, driven by a vision of end-to-end automation which aims to substantially improve efficiency, consistency, and re-usability across the clinical research data lifecycle.
Conferences are a buzz with debate on the best languages to use to implement this future vision – will SAS prevail? or open-source languages like R or Python?..
Addressing the challenge of automation by talking about programming languages is like looking at the Moon with a microscope!
Programmer Proverb
This article aims to look at the heavens using a telescope!.. OK, enough with the analogies!
Let’s start by trying to understand what exactly the challenge is with automation?
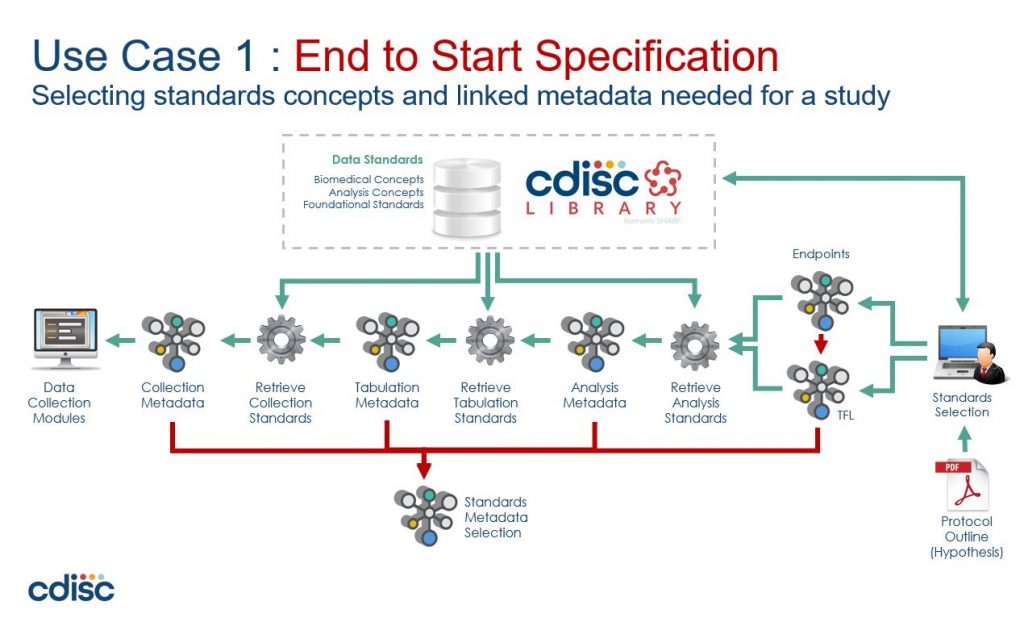
Trouble in paradise
Lets consider a typical automation project:
First, you build a proof-of-concept – maybe an R-Shiny front-end onto a SAS backend that re-uses macros that you’ve been using for years. Everything looks good, ‘click-a-button’ demos, great powerpoints, and everyone is excited… so onto the next stage…
Next is a pilot project – you need something more robust (did someone mention validation??).. Build a web interface in Python, put the metadata in a (graph?) database with the remnants of the PoC (which has now been tested) driving the SAS backend.
Perfect! Now you can see the benefits of “substantially improved efficiency, consistency, and re-usability”.. Can’t you?
What do you mean no? Why not?
Well, because there still seems to be a lot of manual work managing all that metadata.
The problem is that while we no longer have to manually create and configure a suite of programs, now instead, we have to manually create and configure all the metadata that drives the automation.
So, the challenge of automation is actually the challenge of how to efficiently manage metadata.
The tower of Babel
So, lets now examine the source of this inefficiency. We can trace it back to a couple of key assumptions:
- A clinical trial involves many specialist roles – Investigators, Clinicians, Regulators, Data Managers, Statisticians, etc. Each with their own definitions and terminology – their own domain-language. The assumption that a single common data model can fit all these specialist sub-domains results in a data model that is unwieldy, hard to use and even harder to change.
- The assumption that metadata must be stored in a central metadata repository (MDR) leads to a ‘database-centric architecture’ which is problematic in its own right, and also has increased cost of ownership (e.g. managing and maintaining the database server) – not to mention the ubiquitous form-based user interface that needs an endless series of click-and-wait manual steps for every task
These assumptions lead to a ‘tower of babel’ scenario where we have a common data model that is stretched in every direction trying to support every nuance of every domain-expert, and is stored in a central database which becomes increasingly inefficient to use and manage as it gets wrapped in IT processes and change requests.
What we need is an ‘de-centralised’ approach that does not have a single common model in a central repository
Confound their language
To explore what such a ‘de-centralised’ approach might look like, let’s walk through an example using the BRIDG model
The goal of the BRIDG Project is to produce a shared view of the semantics of a common domain-of-interest, specifically the domain of basic, pre-clinical, clinical, and translational research and its associated regulatory artefacts.
BRIDG Model website.
https://bridgmodel.nci.nih.gov/
The diagram below shows the BRIDG model in its full glory. If this one diagram does not communicate the complexity of a single domain model then nothing will!
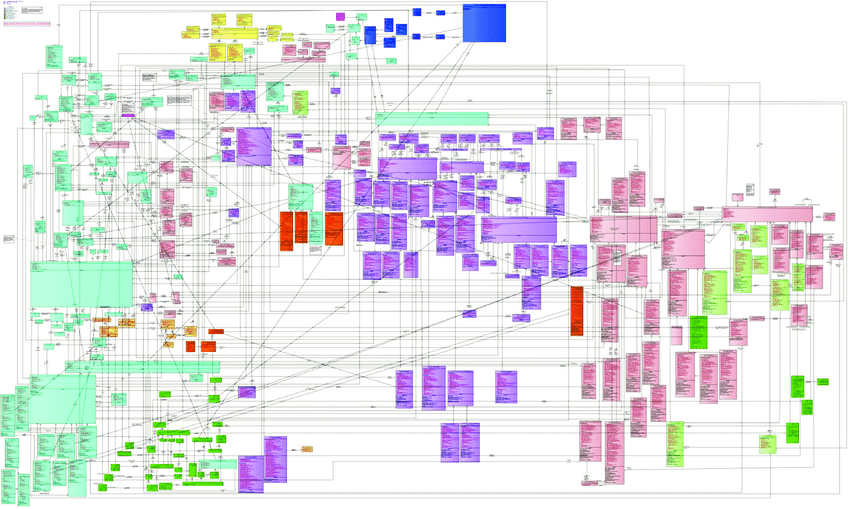
https://cbiit.github.io/bridg-model/HTML/BRIDG5.3.1/
To be fair, BRIDG make it clear that this comprehensive view is published to check model integrity, and users are recommended to use the sub-domain views to understand the model.
The fact that BRIDG recommends sub-domain views confirms the complexity of a common model, but also identifies a solution – simply split the common model into parts!
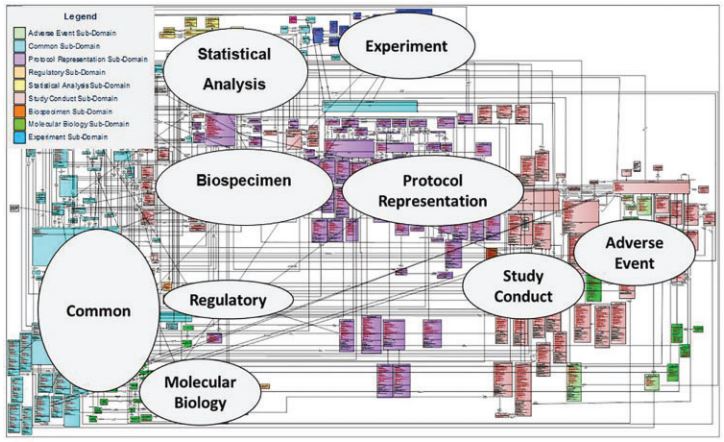
https://pubmed.ncbi.nlm.nih.gov/28339791/
The annotations in the diagram above are simply drawn onto the image – they are not part of the model, do not describe the relationship between sub-domains, and are not machine readable.
The BRIDG model is written in Unified Modelling Language (UML), a general-purpose modelling language intended to provide a standard way to visualize the design of a software system.
What we need is a “meta” language that sits “above” UML, to describe component parts and relationships between these parts.
Positive space
More specifically, we want to use this “meta language” to:
- Model different sub-domains of the clinical trials lifecycle that have specific terminology and definitions used by domain expert working within that sub-domain, because e.g. Statistician’s speak a different language than Investigators, or Data Managers, etc.
- De-couple sub-domains and describe relationships between them and how they are shared and translated. For example both the Experimental and the Statistical Analysis sub-domains have the concept of an unscheduled visit, but each treats them differently, however we still want to link and translate between the two.
Great – so now we know what we want to do with our “meta language”.. but what is it?…

A pattern language
A pattern language is an organized and coherent set of patterns, each of which describes a problem and the core of a solution that can be used in many ways within a specific field of expertise.
https://en.wikipedia.org/wiki/Pattern_language
The term “pattern language” was coined by architect Christopher Alexander in his 1977 book A Pattern Language.
Although the original concept came from building architecture and urban planning, pattern languages have since been used in many other disciplines – and since the 1990s for software design patterns.
One such software pattern language is Domain-Driven Design (DDD), a term coined by Eric Evans in 2003 in his book of the same name.
Domain-Driven Design
Domain-driven Design is a software design approach focusing on modelling software to match a domain according to input from that domain’s experts.
https://en.wikipedia.org/wiki/Domain-driven_design
Well, that sounds promising! Let’s see if we can apply Domain-Driven Design to the BRIDG model…
DDD uses context maps to describe how a domain is divided into parts and the relationships between those parts.
Consider the BRIDG context map below, which was generated from a description written in the Context Mapper language. Notice how this resembles the informal annotations on the UML diagram above.
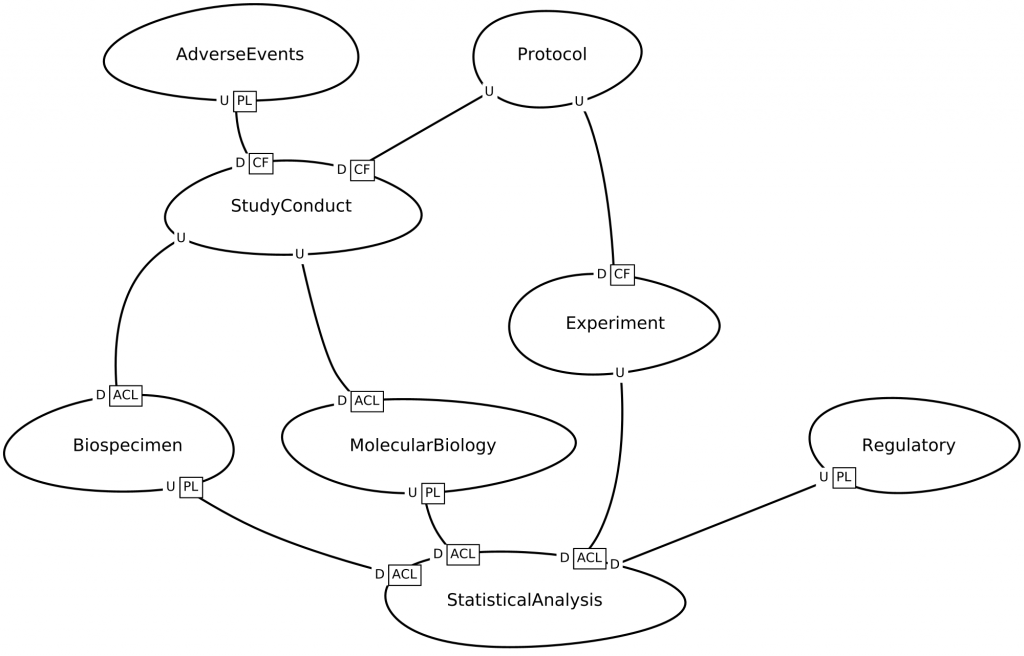
However, this context map is fundamentally different from the annotated UML diagram above, because:
- This context map is generated using a domain-specific language (DSL), which means that the pattern language is an actual ‘computer language’ that can be used in programming editors like VS Code or Eclipse, etc. with syntax highlighting, code-completion, etc.
- Not only can the DSL be used to generate diagrams, it can also be transformed into other models – for example into microservice contracts which in turn can be used to generate code.
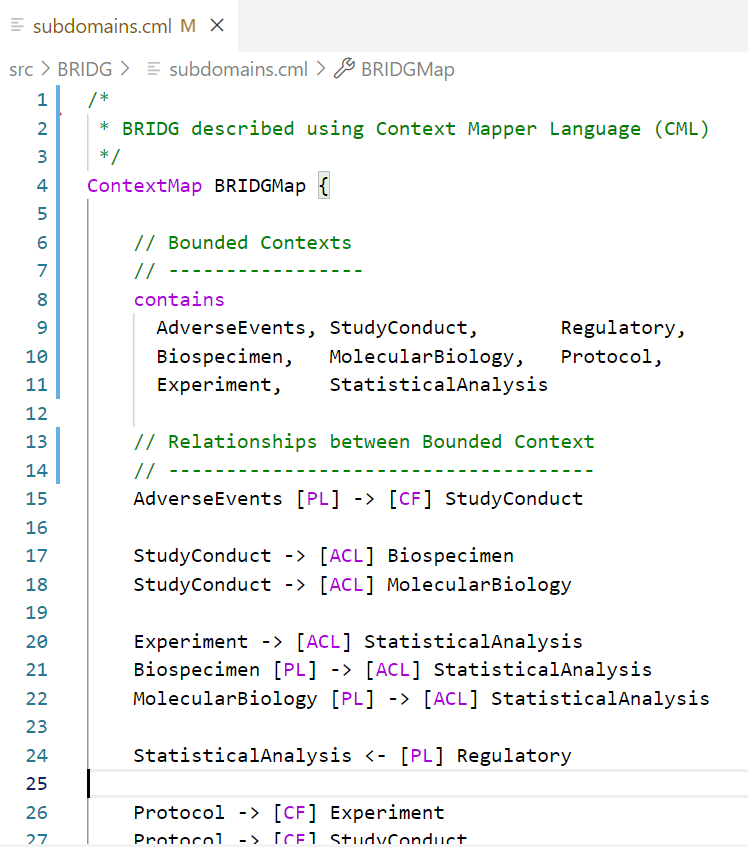
So there we have it! A pattern language that allows us to pull down the Tower of Babel.
- We can create models of the clinical trials domain split into component parts, and model those parts independently while managing the interfaces between them. So, we don’t need a single common data model.
- We can treat metadata like program files – stored in text files that are ‘linked’ together when they are ‘run’. So, we do not need a central database to store metadata.
- Metadata is machine-readable and can be transformed into different metadata files, diagrams, or program code that processes clinical data. So, we can automate end-to-end
Summary
We started off by looking for a way to deliver on the vision of end-to-end automation and achieve the benefits of substantially improve efficiency, consistency, and re-usability across the clinical research data lifecycle.
The assumption that we need a single common data model stored in a central repository leads to inefficiencies which negate the benefits of automation.
Pattern languages are commonly used in software engineering for reusable solutions to commonly occurring problems, and we walked through an example by applying Domain-Driven Design to the BRIDG model.
Using a domain-specific language (DSL), we are able to split the common data model into parts, and store each part as machine-readable metadata in text files to generate code.
This approach streamlines metadata management because we can leverage source-code processes and tools to manage metadata – syntax checking, version control, unit testing, CI/CD, etc… and not a web-form in sight!
Stay tuned for more examples and demos which are in the pipeline.. in the meantime here is some background reading: